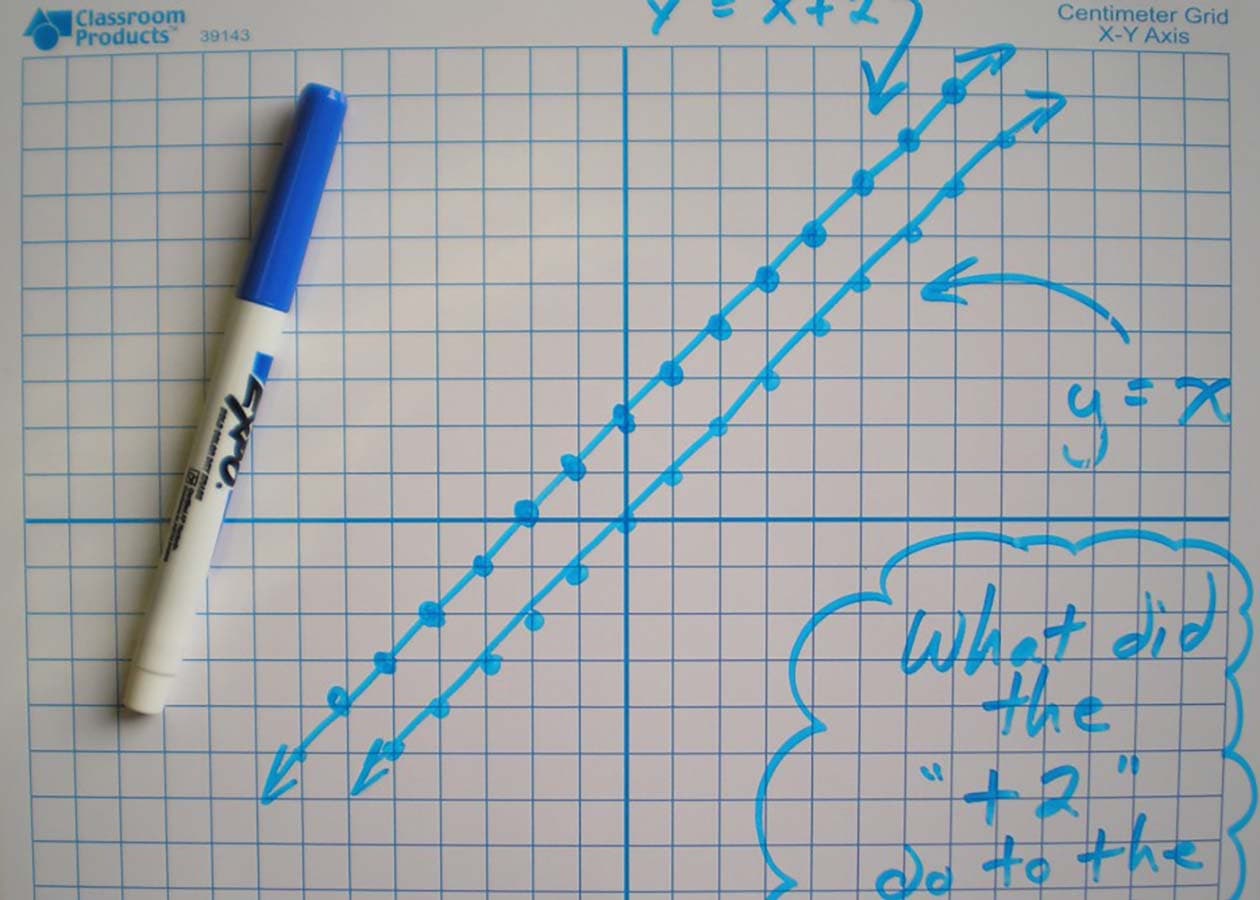
Bottom line: "Indeed, beyond all those statistical models, getting the right users is sometimes as important as (if not more important than) getting enough users." In other words: test users, enough users, but most importantly, the right users. And don't fret too much over figuring out exactly how many users. You'd be missing the point.
The EchoUser research team had quite a busy December. Our schedules were filled with recruiting users, drafting test plans, moderating usability sessions, writing reports, and, last but not least, arranging check-in meetings with clients throughout the project cycle. Clients — regardless of their UX background — would raise questions and concerns about UX methodology in those meetings to make sure that their studies were on the right track and that they would get valuable and defensible data from the projects. In the two usability projects I am on (both benchmark studies), I came across the following two interesting questions from our clients. Though the two questions seemed to have come from two different angles, they both point to one of the key issues in doing usability studies: how to interpret usability data with a small number of users. I thought I’d share the two client questions and hope to elicit some extended discussions here.
Client Question 1: How many participants is enough for a benchmark usability study? Eight, 10, or 12?
A lot of times, the question actually becomes, “Do we need a single-digit participant number or a double-digit one?” Clients want the usability study results to be defensible both from a statistical and a PR standpoint. When time and resources allow and it’s easy to recruit target participants, the question of “Should we get two more participants for the study?” has an easy solution: Let’s just do two more sessions. However, in a scenario in which qualified participants are very difficult to find or recruit (for instance, the study requires a highly specific user profile) or time and resources are limited, how many participants are needed? Is it worthwhile to spend two more weeks on the study just to make it to a total of 10 participants? The bigger issue: What is the rationale we should use to validate the number of participants for a usability study? If we go back to the classic model from Nielsen, five users are enough to uncover 85% of usability issues. That has been the UX industry standard’ for a long time, as Jakob Nielsen and his colleagues were among the first UX professionals to calculate the relationship between the number of UX issues uncovered and the number of participants involved. The mathematical model is derived from their years of experience conducting usability studies. Faulkner challenged Nielsen’s model in 2004 with a paper named “Beyond the five-user assumption: Benefits of increased sample sizes in usability testing.” She carefully designed and conducted a few studies with different sample sizes (5, 10, 20, 30, 40, 50, and 60 participants). What she learned from the follow-up data simulation and analysis is that 10 participants are enough to identify at least 82% of the usability issues, whereas a sample size of 15 can help to identify at least 90% of the issues. I even came across a sample size calculator on Jeff Sauro’s Measuring Usability site. Based on the binomial probability formula, it allows you to calculate, for instance, how many users are needed to discover 80% of the usability issues when all issues’ probability of occurrence is above 30%. All of the above can be used as reference rationales to validate using a certain number of participants for a study. However, as specifically mentioned in Faulkner’s paper,
Having a highly representative user sample is crucial in uncovering the priority usability issues. Indeed, beyond all those statistical models, getting the right users is sometimes as important as (if not more important than) getting enough users.
Client Question 2: Are we telling the product team that 80% of our customers will fail to use this functionality because 8 out of 10 users failed in the usability study?
Well, the primary purpose of usability studies is to discover qualitative usability issues with an interface, as opposed to predicting the probability of those issues’ occurrence. However, the task completion rate is one of the key metrics we use to evaluate the usability of different UI features, and it is our responsibility to give clients and the product team a clear idea of how to interpret the completion rate. The confidence level of the results is, again, closely related to the number of users included in the study. From a statistical standpoint, it’s not difficult to understand that the more users in the study, the more confident we can be in the results. However, with only 10 participants, how confident can we say we are in our results? John Sorflaten has an interesting article discussing this topic. He put forward the limitation of using task success data to predict customer behavior on a larger scale. He recommended using the Adjusted Wald Interval calculator coded by Jeff Sauro to generate the lower and higher bounds of the task success data. For instance, if 8 out of 10 participants succeed in a task, how could this data be used to predict 1,000 or 10,000 users’ behavior? By using a confidence level of 95% (if you run the same test 100 times, 95 of the times the results will fall within the acceptable +/- margin), Jeff’s calculator generates a lower bound of 48% success and a higher bound of 96% success based on the 80% task success rate from the usability study and accounting for the small sample size. And the same is true if 8 out of 10 participants fail in a task: The calculator predicts a chance of as few as 48% or as many as 96% of users failing the task when the UI is actually released and on the market. In that sense, as opposed to using the 80% task success rate to predict broader user behavior, we as usability professionals can show the range between 48% and 96% as a reference range for the product manager or marketing team to make further interpretations or decisions.
Next time, when clients are debating between 8 or 10 participants, or the product manager is asking why the task completion rate does not match large-scale user data, these basic stats will help to answer the questions.